Dissatisfaction with democracy is at a high. In 2020, a report by the Centre for the Future of Democracy at the University of Cambridge showed that 58 per cent of people living in democracies are dissatisfied with their democratic institutions (up 10 percentage points since 1995, when this data was first reliably tracked). This rise in dissatisfaction is especially pronounced in developed countries such as the United States (55 per cent dissatisfied, up 30 percentage points vs. 1995).
Dissatisfaction with democracy (% of all respondents)
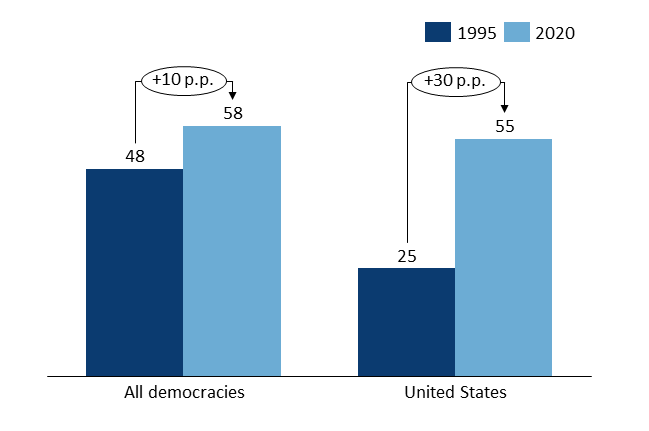
Source: University of Cambridge’s Centre for the Future of Democracy
To govern effectively, democratic leaders and institutions must be perceived as legitimate. They must be trusted. Be chosen, committed to.
I don’t have the answer on how to resolve this. There likely isn’t One Answer. What I propose is that we experiment – broadly, quickly and ambitiously – with ways to help us regain confidence in our institutions.
One obvious line of experimentation is to change the mechanisms used to set our laws and policies. Another is to improve the quality of the customer experience delivered to citizens. In what follows, I’ll make the case – and try to flesh out the practical elements to get right if you want to try this – for incorporating prediction markets into how we govern to increase legitimacy. Bear with me.
In today’s democracies, voters elect leaders to represent their preferences. These leaders represent both values about what to do and beliefs about how to do it. More than 20 years ago, economist Robin Hanson proposed a new governance system that disaggregates these two factors by letting constituents “vote on values, but bet on beliefs”. In this system – which he called futarchy – democracy is used to decide what we want, and prediction markets are leveraged to determine how best to deliver it.
In the past two decades, futarchy has not gained widespread use. But … there is better knowledge about when and why prediction markets work (common examples include betting odds for elections and sporting events). Largely through advances in fintech and crypto, people have also gained interest and experience interacting directly with financial markets. As the world gets more complex, the expertise required to govern has become more and more distributed. I therefore believe it is time to reassess where and how prediction markets could improve governance.
Chapter 1
A prediction is the expression of a belief about how the world will be. We live in an uncertain world, which we observe imprecisely. To navigate it, we constantly make predictions. We predict the weather, commuting time, how a work email will make a colleague feel, the future price of a stock. Prediction markets enable us to aggregate these beliefs by enabling people to bet on a question of interest.
At a minimum, the question of interest should be time-bound – predictions should have a clear resolution date – and should also be resolvable: the outcome of the prediction should be objectively determinable, with clear rules agreed in advance.
At the time of writing, the most popular question on the academic prediction market PredictIt is “Who will win the 2024 Republican presidential nomination?” (for those interested, Donald Trump is leading as of 12 December 2021. A share that will pay out $1 if he wins is currently trading at 41 cents. This price can be interpreted as traders’ aggregate belief that he has a 41 per cent chance of winning the election). This question is time-bound and resolvable. We know that the Republican party will nominate a candidate in advance of the 2024 presidential election.
Chapter 2
Prediction markets have a proven track record of providing accurate forecasts of uncertain events (see Snowberg, Wolfers, and Zitzewitz’s 2012 working paper for a good overview).
Politics
The Iowa Electronics Market (IEM) – operated by academics from the University of Iowa – has been running election prediction markets since 1988. The IEM has been shown to outperform pollsters. For example, over the run-up to the election, its markets have half the forecast error of polls and have made correct election calls 451 out of 596 times. These results are not unique to the IEM: other prediction markets have achieved similar performance.
Business
Corporations have also successfully leveraged internal prediction markets to forecast key operational variables such as future sales or the time needed to complete a project. For example, prediction markets at Google, Ford and a basic materials conglomerate have been shown to improve upon expert forecasts by as much as 25 per cent. Eli Lilly, General Electric, France Telecom, Hewlett-Packard, IBM, Intel, Microsoft, Siemens and Yahoo have also successfully leveraged prediction markets to improve their internal forecasts.
Decentralised Autonomous Organisations (DAOs)
Decentralised autonomous organisations (DAOs) are a new type of organisation applying advances in blockchain technologies to governance. As of 2020, at least one of these organisations – Gnosis – is leveraging the principles of futarchy to govern itself.
Chapter 3
Prediction markets could create value by helping us govern better. After choosing goals using a democratic process, prediction markets could improve on our institutional ability to achieve these goals. Below I will describe three potential applications of prediction markets. Examples have been ordered to illustrate increasing degree in market design complexity. The section gets a bit technical – please skim the implementation details if they’re less relevant to you!
Some of the use-cases explored include responding to crises better, choosing between different interventions to reach a goal, and setting long-term policy guidance like budget rules or monetary policy.
Crisis Response: Determining Optimal Mask Policy for Covid-19 in March 2020
The Covid-19 crisis required governments to make important decisions, under significant uncertainty, quickly. This is difficult.
Prediction markets could have made this easier. For example, let’s revisit mask mandates. In March 2020, the Centres for Disease Control and Prevention (CDC) in the United States recommended that the general public did not wear masks to protect against Covid-19.
While countries like Germany and the United Kingdom had similar guidance, elsewhere, such as in China, Hong Kong and Japan, authorities recommended wearing masks, especially in crowded places.
The recommendation not to wear face masks appears to have been driven by the lack of studies “proving” that masks did reduce community transmission. While institutions like the CDC are culturally risk averse, speculators on a prediction market would likely not have suffered from this bias.
A savvy government could have leveraged a prediction market to quickly pool the best perspective on the effectiveness of mask mandates for the general population. In fact, a similar question was asked by superforecaster Michael Bishop on the prediction platform Metaculus.
For example, they could have asked, say in February 2020:
In February 2021 (in one year), will the CDC evaluate that “endorsing mask wearing” starting in March 2020 would have been a superior policy to “recommending that Americans do not wear masks”?
“Endorsing mask wearing” is defined as recommending that all American adults wear cloth or medical masks in indoor shared spaces like stores and public transit to protect against Covid-19.
“Recommending that Americans do not wear masks” is defined as recommending that American adults do not wear masks in shared spaces like stores and public transit to protect against Covid-19.
As described below, this prediction market could have been implemented in a cost-neutral way by the CDC (or another actor).
Step 1: Issue securities for the question of interest to create the prediction market
Issue two securities for the question of interest. One security – which we will call the “yes” security – pays $1 if the CDC concludes in February 2021 that endorsing masks in March 2020 would have been the best course of action to limit Covid-19 transmission. The other security – the “no” security – would have the opposite payoff, paying $1 if the CDC does not retroactively conclude that endorsing masks would have been effective. To be cost-neutral, the CDC would issue these security pairs for $1 total.
Step 2: Let participants trade the “yes” and “no” securities
The issuer should then let the “yes” and “no” securities be traded freely. In each market, the trading cost of the “yes” security would represent the best market estimate of the probability that the CDC would retroactively declare that endorsing masks was (or would have been) the optimal policy choice to reduce Covid-19 transmission. For example, if this security is trading at 80 cents, it is believed that the CDC has an 80 per cent chance of concluding that endorsing masks would have been effective in reducing Covid-19 transmission in March 2020 (that is $1 if the goal is achieved multiplied by an 80 per cent chance of being achieved equals 80 cents).[_] The CDC could then act on the prediction market’s knowledge and update its recommendation on mask wearing.
Step 3: Resolve the market by measuring results and paying out the “yes” or “no” security
In February 2021, the actor would pay out the “yes” security if the CDC had concluded that endorsing masks starting in March 2020 would have been more effective than recommending against mask wearing. Otherwise, it would pay the $1 to the holders of the “no” security. By having issued both securities, this payout would come at no cost to the actor who created this market.
Illustration of an implementation of a prediction market
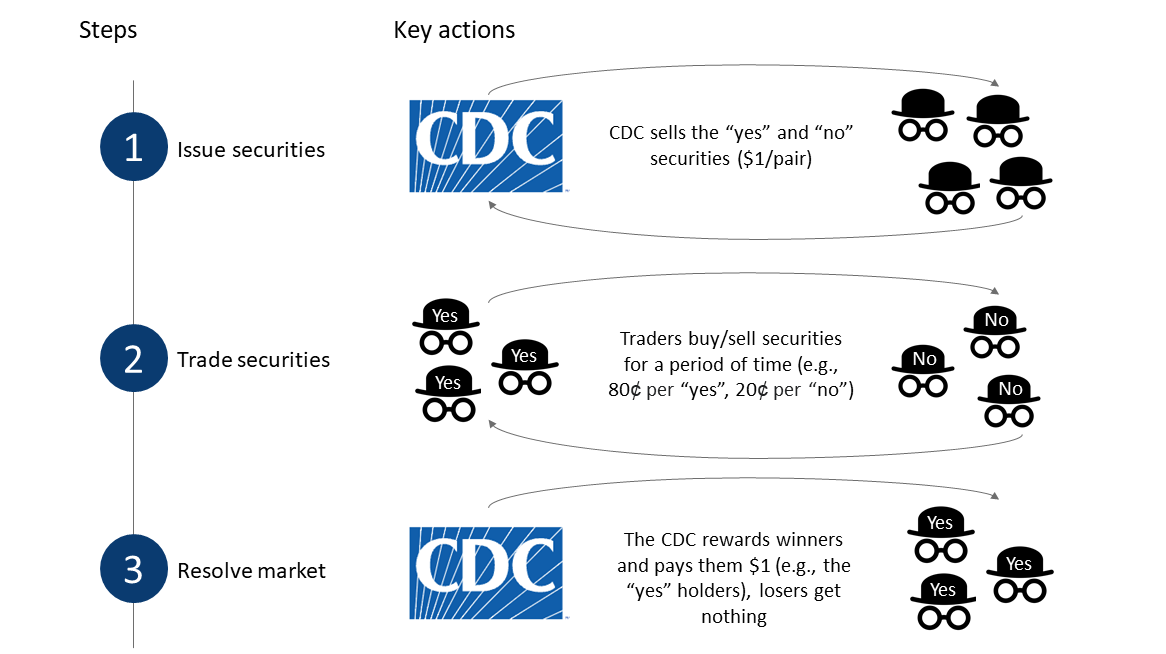
Source: Marc-André Simard
Additional Thoughts
This is the simplest design for a prediction market. It requires market participants to bet only on one specific question. While the question is self-referential (in other words, it asks for a prediction about the CDC’s perspective on current events in a particular year) instead of asking for an objective assessment of the effectiveness of masks (which could take years to arbitrate, especially if the evidence is mixed), it is simple and ensures a decisive resolution to the question. This practical approach to using prediction markets has the advantage of being deployable quickly in a crisis. More complex implementations will be discussed below.
Intervention Selection: Choosing an Intervention to Improve Literacy Test Scores
Around the world, governments invest significantly in educating their young. Where I’m from (the province of Ontario in Canada), 72 local school boards receive funding from the provincial government to educate children according to a standardised curriculum. Ontarian students complete reading, writing and mathematics testing in grade 3, grade 6 and high school.
While school boards have constraints on how to deliver the curriculum (for example, maximum student-teacher ratios and set school calendars), they have discretion on how to spend a meaningful portion of their budget.
Let’s assume that one of the school boards wants to improve their high school literacy scores for the upcoming academic year. While they have shortlisted three potential interventions, they are limited to the expertise – and credibility – of their existing (often small) team of educators to select the best path forward. Specifically, they have shortlisted three programmes in which to invest $10 million of discretionary funds with the goal of achieving at least half a standard deviation increase in average test scores:
Providing daily one-on-one tutoring to the lowest-performing students
Providing weekly one-on-one tutoring to all students
Paying students based on their literary test results
They could use a prediction market to aggregate knowledge – across a much larger talent pool – to decide on the best path forward. Specifically, they could ask:
“Which intervention (A, B or C) would be most likely to improve average test scores by half a standard deviation by the end of the 2022 school year?”
This prediction market design is a bit more complex. It leverages conditional prediction markets – asking traders to forecast an outcome if and only if a certain action is taken – to get a prediction if different interventions are put in place.
Step 1: Create three prediction markets: one for each intervention
Three markets – one for each potential intervention – could be created. For each market, two securities should be issued. One security, which we will call the “yes” security, pays $1 if literacy scores are increased by half a standard deviation, conditional on a specific programme being implemented (for example, providing one-on-one tutoring to students whose performance is in the bottom quartile), and $0 otherwise. The other security – the “no” security – would have the opposite payoff, paying $1 only if the intervention is not successful in raising literacy scores by half a standard deviation. By issuing securities with opposite payoffs, the school board would ensure that it would be able to create this market at no cost to themselves (the market is zero-sum; winning participants are only compensated by losing participants).
Step 2: Let participants trade the “yes” and “no” securities on each of the three markets
The school board should then let the “yes” and “no” securities be traded on these three markets freely for a set period of time – say the two-month summer break. In each market, the trading cost of the “yes” security would represent the best market estimate of the probability for the intervention (if implemented) to raise literacy rates by at least half a standard deviation. For example, if this security is trading at 70 cents, it is believed that the intervention has a 70 per cent chance of reaching the literacy goal (in other words, $1 if the goal is achieved multiplied by a 70 per cent chance of it being achieved equals 70 cents).
Step 3: Implement the policy with the highest “yes” price, void all other markets
At the end of the trading period, the school board should commit to implementing the policy with the highest “yes” price. This is the policy most likely to increase literacy test scores by half a standard deviation.
It should then void all transactions on the two markets with lower “yes” prices, as their policies will not be implemented, and the bets will therefore not be resolved. Trading on the market that has not been voided could continue to provide liquidity to participants who want to exit their position and lock in some profits.
Step 4: Measure results and pay out the “yes” or “no” security
At the end of the school year, after the intervention has been implemented and standardised tests have been graded, the school board will be in the position to assess if it has reached its goal. If literacy scores have been increased by half a standard deviation, it pays out $1 to all holders of “yes” securities. If it hasn’t, the money is paid out to holders of the “no” security.
Additional Thoughts
In step 3, if all “yes” securities have very low prices (e.g. below 20 cents), it is unlikely that any of the interventions would be successful in reaching the school board’s literacy goals. At this point, the school board could also decide to void all prediction markets and go back to the drawing board.
The conditional prediction markets enable the school board to gain insights on different potential futures, depending on which action it takes. This provides richer information, at the cost of adding complexity (which could reduce participation by traders).
Structural Policy-Setting: Selecting the Interest Rate of the Central Bank
Similarly, prediction markets could be used to gain insights about macro policy decisions such as how much fiscal stimulus to deploy during a crisis or on optimal monetary policy.
One way to implement prediction markets to guide the selection of the interest rate of the central bank would be to have three conditional markets (as in the education example above), running each week (or month). Prediction markets would forecast an outcome variable if the interest rate were increased, decreased or held constant. This outcome variable could be, for example, the average GDP of the country (or a composite index of the GDP and other factors of interest such as the Gini coefficient or the unemployment rate) over a ten-year period. Robert Hanson provides a full treatment of this scenario in his original paper on futarchy.
The wrinkle worth discussing here is that bettors must be incentivised to bet on an outcome that will be resolved over a long period of time. To make it worthwhile, the returns of this prediction market must rival other investment opportunities. One way to provide this incentive would be to pay out in a market index (for example, an S&P 500 ETF) or via a government bond rather than in dollars.
Table Stakes: What You Need to Get Right
Prediction markets aggregate information from its participants. To be successful, attracting bettors is key. This section offers five key factors for building an attractive prediction market in the real world. It has been largely influenced by Zvi Mowshowitz’s article “Prediction Markets: When Do They Work?”
Well-Defined Question and Resolution
A well-defined question ensures that traders can focus on predicting the outcome of the bet, and not on how the question will be resolved. A well-defined question should have clear rules on how it will be resolved, including all edge cases. For example, if betting on “team A will win a hockey match”, resolution should be pre-determined if the game ends in a tie or is cancelled (e.g. team A did not win, or the market is void).
Low Transaction Costs
Transaction costs include fees to fund a betting account, make a trade or withdraw funds. If transaction costs are too high, participation will be limited.
Short Resolution Time
All else being equal, bettors will prefer markets that resolve quickly. There is an opportunity cost of locking in funds in a prediction market. As described in the example concerning central bank policy above, this can be at least partly resolved by enabling bets to be made in an interest-bearing asset such as a stock index or a bond. This way, at least your potential winnings are not inflated away!
Sources of Disagreement or Interest
Without subsidies, prediction markets have “zero-sum” payoffs. This is similar to a game of poker. To incentivise participation, bettors must either believe they have some unique information (they are a better poker player) or want to hedge against a particular outcome. For example, in our first example concerning mask recommendations, if you were a mask manufacturer you might bet against the CDC finding masks useful to buy insurance against your capital investment to scale up mask production. Natural hedgers add liquidity to the market, incentivising others to do research, uncover information and participate. Perhaps counter-intuitively, having participants that are betting for other reasons than finding the right “answer” improves the quality of the outcome by attracting sophisticated investors.
Trusted Market Operator
To attract large sums of capital (and, subsequently, information), the market operator must be reputable. It must credibly be able to enforce the rules of the prediction market. It must not add additional risk of, for example, default or fraud.
It is a good rule of thumb to design the prediction market as simply as possible. Participation can also be increased by subsidising the market (e.g. reimbursing transaction fees, or increasing the payoff to winners at resolution time). One final recommendation is to keep some budget to market the prediction market broadly. The insights will only be as powerful as the collection of perspectives from the participants.
Additional Risks and How to Mitigate
As with most good things in life, launching a prediction market to improve governance is not risk free. Two main risks should be understood and mitigated.
Market Capture by a Policy Interest Group
In a market lacking liquidity, an interest group could potentially come in and change the price of the bets to resolve in its favour. For example, in our first example, a group that was ideologically opposed to a recommendation in favour of wearing masks could aim to influence the resolution of the market.
There are two major ways to guard against this failure. First, liquidity in the market should be encouraged by ensuring that the market has a well-defined question, a short resolution time and sources of disagreement or interest. The market could also be directly subsidised to increase liquidity. With enough liquidity, it becomes cost-prohibitive to capture the market (since people betting against the interest group are getting “free” alpha).
Second, if decisions are made based on the closing price of a market, the bet should not be resolved at an instant in time (e.g. the last second before the resolution date) as this could make it easier for an interest group to swing the market (cheaply) at the last minute. Instead, an average of the betting prices should be taken over a certain period.
Coercion of the Party Resolving the Bet
If the market resolution criterion requires judgement (as in our mask-wearing example), it is conceivable that an interest group would coerce or bribe the party resolving the bet to decide in its favour.
To mitigate this risk, outcomes should be measurable as impartially as possible. As with insider trading on stock markets or match fixing in sports, regulators should also make it illegal for bet resolvers to cheat.
Finally, markets with a high risk of coercion of the decision-maker will tend to reduce the number of traders interested in the market. This should naturally push organisations leveraging prediction markets to design them soundly. It also naturally acts to reduce the incentive to cheat if they are interested in benefiting from this tool in the future.
Perverse Incentives
Traders could also influence the outcome of interest directly to increase the odds that their prediction is materialised. In some cases, this would be detrimental. For example, in our education example, traders betting that test scores will not improve could have an incentive to bribe a teacher to teach poorly or even bribe students directly to perform poorly on the test. If the risk of perverse incentives is too high, it is possible that the question is ill suited for prediction markets.
Chapter 4
While historically illegal in many jurisdictions, prediction markets are gaining legitimacy. For example, in the United States, Kalshi received approval from the Commodity Futures Trading Commission (CFTC) in November 2020 to be the “first regulated financial exchange dedicated to trading events contracts”. Its public beta became live in July 2021, and it is now possible to trade on markets ranging from Covid-19 to politics, economics and climate. While this is a good start, there is much to do to prove the value of prediction markets in improving governance.
Governments
In jurisdictions where prediction markets are legal, governments of all levels could directly experiment with prediction markets to get insight on policy questions where outside expertise would significantly improve their judgement. An ambitious political party could also use prediction markets as part of the process to define their platform prior to an election.
In jurisdictions where prediction markets are illegal or suffer from regulatory uncertainties, governments could make them fully legal. Various avenues exist to do this: prediction markets can be legalised as financial securities or as gambling products. If this is too difficult, as a first step, special permits could be issued to enable organisations to experiment with this technology.
Philanthropists
If governments move slowly on this opportunity, philanthropists could also test the application of prediction markets to governance. For example, a donor could choose a jurisdiction where these types of markets are legal (for example, the US), team up with an existing platform such as Kalshi, and market and subsidise (conditional) prediction markets on pressing policy questions.
The philanthropists could concurrently work with – or at least influence – policymakers with the results of these prediction markets.
Other Organisations
Prediction market operators (or, in jurisdictions like the UK with more liberal betting laws, gambling sites such as bet365, William Hill and Betfair) could independently decide to create and promote markets that could provide helpful guidance to policymakers.
Private-sector companies could sponsor prediction markets on questions of interest to their firm, to further prove the concept. Non-profits could do the same.
Finally, journalists could also incorporate prediction markets in their work, as showcased by Metaculus in their recently launched journal, increasing the adoption of prediction markets as a mainstream source of insight.
Futarchy is unconventional. It is therefore best to start small, learn and improve on our implementations. Best practices for prediction markets should be applied and risks should be mitigated. Most importantly, democratic governments and their supporters should signal their willingness to experiment and rebuild trust with the public. Limited downside, huge upside. That’s a bet worth making.
Thanks to Michael Bishop, Rafael Proença, Ali Schwabe, Nathan Young and Haiyang Zhang for reading drafts of this piece.
Lead Image: TBI